Dobb·E
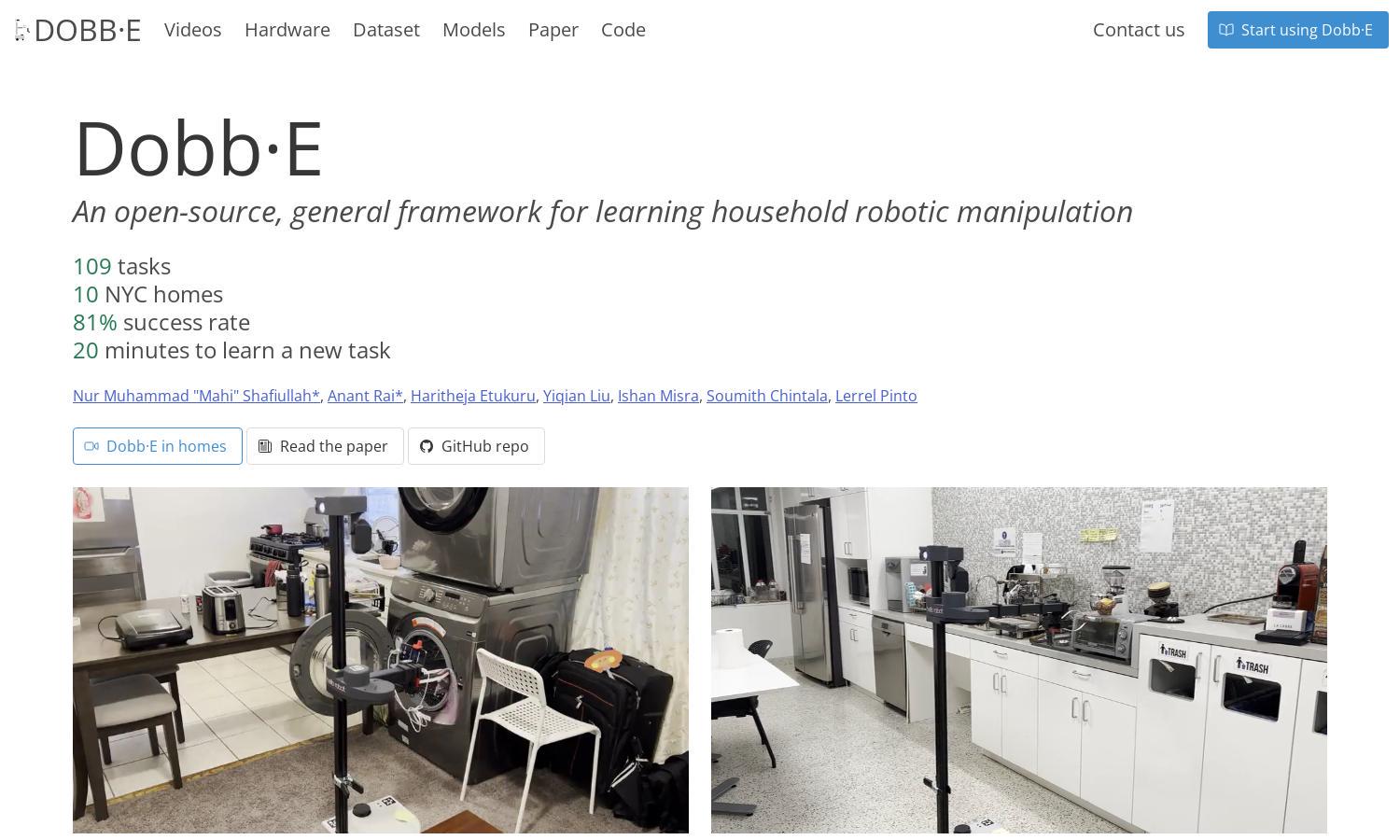
About Dobb·E
Dobb·E is an innovative open-source framework aimed at revolutionizing home robotics. It teaches robots household tasks quickly by leveraging a unique tool, "The Stick," and a comprehensive dataset from New York homes. Users can efficiently train robots with an impressive success rate, enhancing domestic automation.
Dobb·E offers users a completely free open-source platform, eliminating barriers to accessibility. As it evolves, potential premium services might be introduced to enhance user experience further. Gaining access to advanced features may motivate users to upgrade, maximizing their home robotics capabilities with Dobb·E.
Dobb·E features an intuitive user interface designed to simplify interaction with its robust tools. Users can effortlessly navigate the platform, enjoying features like data collection and task training. This seamless experience ensures that even novices can harness the full potential of Dobb·E with ease.
How Dobb·E works
Users interact with Dobb·E by first utilizing "The Stick" to collect demonstration data of household tasks in their own environment. This data, drawn from 13 hours of interactions across 22 homes, is then processed to train the Home Pretrained Representations (HPR) model. Subsequently, users can apply this training, adapt it within a new home setting, and see robots learn tasks efficiently, all while enjoying an 81% success rate.
Key Features for Dobb·E
Imitation Learning Efficiency
Dobb·E stands out due to its efficient imitation learning capability, allowing robots to acquire new household tasks precisely. By using minimal demonstration time and leveraging the Homes of New York dataset, Dobb·E enhances user experience in home robotics and accelerates the path towards practical domestic automation.
The Stick
Dobb·E features "The Stick," a cost-effective tool for collecting demonstration data efficiently. By enabling easy and ergonomic demonstration gathering, Dobb·E empowers users to train robots effectively, highlighting innovative user-centric design that breaks down barriers in household robotics, enhancing learning capacity.
Home Pretrained Representations (HPR)
Dobb·E utilizes Home Pretrained Representations (HPR) to initialize robot policies quickly. This powerful model, pre-trained on extensive datasets, ensures robots adapt faster and more efficiently in novel environments, significantly improving their capability to perform varied household tasks successfully.
You may also like:
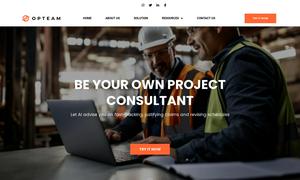